Creating a digital twin of manufacturing operations can deliver development and efficiency benefits. Mike Farish reports
Digital twinning allows companies to effectively utilise the data gathered from manufacturing equipment through constructing a comprehensive digital counterpart to a physical installation and the processes carried out by or in it, which can be used to support the operation, simulation and analysis of that installation and its associated processes.
Ultimately the concept envisages situations in which the real-world object and its digital representation are synchronised with each other so that they possess identical interlinked capabilities. In this scenario an operation in the physical world should produce the same effect on its digital twin as upon the real object or process with the reverse being equally true.
Someone for whom the term is of daily relevance is Dr Olaf Sauer, coordinator of the automation business unit of the Fraunhofer Institute for Optronics, System Technologies and Image Exploitation (IOSB) in Karlsruhe, Germany. He indicates that trying to understand the concept is, perhaps, less a matter of formulating a single definition than identifying what it can make possible. “From our point of view digital twins are not single objects or monolithic data models, but are composed of different aspects of digital representations, functionalities, models and interfaces,” he explains. “We are, for instance, currently working on digital twins in an automotive press shop where we model the single processes in detail to figure out the relations between values from quality control and process parameters in the presses.”
Dr Sauer notes that from a manufacturing engineering perspective, it is evident that digital twins perform a number of different tasks, including:
- Self-description using unique attributes and parameters describing configuration data, such as auto-identification, to connect machines and components easily to wider software systems.
- Modelling of the correct runtime behaviour of a machine, a line or an entire manufacturing shop based on data acquired from machine learning.
- Offline and online simulations including virtual commissioning in which produced goods interact with machine kinematics. Ideally, different simulation models must be able to interact to form an integrated simulation model.
- Digital factory modelling describing machines and other manufacturing resources, buildings and utilities. The concept of digital factories already has a long history and is described by well-known standards, such as VDI 4499.
A scalable concept
Nevertheless, a point that Dr Sauer stresses is that in practice the concept is infinitely scalable. “All processes can benefit from digital twinning regardless whether they involve an automated machine tool or a manual assembly procedure,” he confirms. “A digital twin may be for a single machine or for an entire production line.” Similarly, a digital twin may involve the replication of something quite tangible such as a hardware element or of something much more diffuse such as a process. Dr Sauer mentions in this respect an evaluation of the concept from automation supplier Siemens. “They say that the entire digital twin consists of a digital twin of the product, a digital twin of production and a digital twin of the product’s operation,” he states.
In turn that means that the software used to construct a digital twin might be the one that is used to control the real-life operations involved or might instead be a more generic simulation package. “Simulation of manufacturing operations might involve the actual software that will be used in production,” Dr Sauer confirms. “But for a case in which manufacturing is being planned the simulation might not be a real time simulation based on runtime data from operations but a factory planning simulation based on assumptions.”
But Dr Sauer is emphatic that though the concept of digital twinning may sound esoteric it is when properly implemented of real practical value. “Digital twins help to get a better understanding of processes and can also be used for predictive applications, for instance in the monitoring and control of complex production lines,” he states. “Imagine that a monitoring system collects data from the PLCs. The data may then be used to run an online simulation to support the operators in cases where a machine has a breakdown. Based on the runtime data the simulation would be able to predict the breakdown’s impacts on connected lines of resources such as conveyors and other equipment.”
The take-up of the approach so far, however, is still limited and the potential for the further implementation correspondingly extensive. “According to Gartner today less than 1% of physical machines and components are ‘modelled such that the models capture and mimic behaviour’,” Dr Sauer reports. “So there seems still a lot to do to come to a full picture of digital twins.”
But, Dr Sauer continues, what does seem evident from experience so far is that the application of digital twins will be very specific and always according to the use case. Any successful implementation must also be holistic in that all elements of a twinned system must be able to interact with each other. “It must be possible to integrate the different part models of digital twins,” he states.
Practical application and tangible results
Meanwhile Nissan in Sunderland in the North East of England, has been looking to explore how simulation based on the concept of digital twinning can be exploited to support a range of operations. Martin Perkins, industrial engineer at the site, confirms the company has been using a simulation package called Witness Horizon from UK-based software supplier and consultancy Lanner “for over ten years” to help enhance operations including cylinder head machining and electric vehicle powertrain production.
On the first of those counts the system was used to help rationalise the use of pallets that carry the cylinder head workpieces through a process involving primary machining, cleaning and then secondary-stage machining. When faced with the prospect of investing in new pallets Nissan wondered if the facility could operate with a reduced number of pallets without negatively affecting the current throughput rates. A digital twin of the cylinder head machining line was therefore used to run various scenarios for different pallet volumes with variables including inputs, cycle time and volumes. The simulation showed that a stock of 70 pallets would be sufficient to support operation of the process at full capacity – less than the anticipated figure of a hundred. This produced a saving in investment in new pallets of £22,000.
On the second when the company reports that when it was preparing to launch an updated version of its Leaf electric car, featuring a new, more advanced motor the factory needed to double its powertrain throughput, from five jobs per hour to ten. The industrial engineering team therefore wanted to identify potential bottlenecks with an updated production design identifying the process improvements that would be required to facilitate the increased output.
Using predictive simulation, the team identified a crucial potential bottleneck in the sealing process deriving from the fact that once a seal is put on the motor unit it has to cure for 15 minutes before it can be leak tested. Even after modelling several change scenarios, this blockage still could not be eliminated.
The model was used to experiment with various countermeasures. The solution was to implement another loop that provides additional powertrain unit storage capacity which increased throughput while still providing the required cure time before seal testing.
“Simulation is one of wide number of benefits digital twins can offer in addition to improved understanding, enhanced control of resources and automation of business processes,” says Perkins of Nissan’s experience of the technique. “An abstract model of physical assets, process logic and operating features sits at the heart of a digital twin and by combining this with simulation technology enables predictive analysis, experimentation and optimisation of the end-to-end value chain.”
Perkins also says that the type and volume of data needed to drive an effective digital twin simulation process vary according to several factors. “The data inputs and, more specifically, the level of detail at which digital twins need to be modelled depends very much upon the requirements of its users and also how it is intended to fit within a wider digital twin strategy,” he states. “For example, a low fidelity/highly abstracted digital twin may be created for initial line concept design that provides approximate answers to questions such as cost of implementation, expected throughput and labour requirements.”
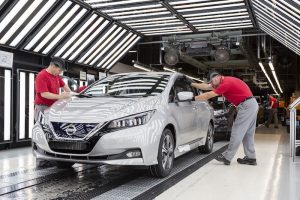
Speed and flexibility in development
Moreover, Perkins continues, the benefits deriving from that initial model can be developed quickly and yet retain the flexibility to evolve. “That same digital twin model may then be enhanced with more detail during real world implementation of the line by connecting it to real performance data such as sensor readings and breakdown statistics in order to optimise process elements such as rightsizing buffers or justifying predictive maintenance policies,” he confirms. “The key to many successful digital twin implementations is that users are able to easily understand its capabilities and have faith in the information they provide.”
Nissan, Perkins confirms, is deriving real benefits from its use of digital twinning simulation. “Witness allows machining operations to be modelled very efficiently,” he states. “It would take just minutes to model several machines of this nature using its native machine element and there are already 2D and 3D objects that fit most operations in the software’s manufacturing pack.
But Perkins also recognises that the further development of the technique for Nissan will involve the use of more powerful analytical capabilities. “Evolving digital twins to the next level, beyond the analysis and insight stage, so that they can control operations directly, for instance by changing PLCs, policies and rates, will require more than our present software and could involve a substantial investment”, he states. One reason for this that Perkins identifies is that “underlying execution systems need integration and event orchestration is needed to enable this from a technology perspective.” But in addition there is also the people factor – the fact that much of a company’s expertise is quite literally embodied in the experience and skill of its employees. “There is a lot of knowledge across labour forces that operate across wide, complex production lines that is not easily replicated by a digital twin and users must be confident that digital twins are capable of delivering correct and beneficial changes before any form of automation can be deployed,” he explains.
But Perkins has no doubts that the benefits of digital twinning are not confined to pre-operational simulations but also extend to its use as a tool to support actual manufacturing. “Once a digital twin has moved the to the operational stages, events triggered by IoT sensors or similar can inform it that a process is not running as expected,” he confirms. “Any impact on production, including delivery times and future bottlenecks, can be identified. The users of a digital twin can then make adjustments to mitigate the problems based on the information they have and the timeframe they have to make a decision.” Ultimately, he continues, “this will eventually lead to the digital twin seeking a range of optimal answers that are either presented to the user to implement or are implemented directly back to the line via a control mechanism following business policies.”
As such Perkins takes the view that the Nissan Sunderland Plant is still in the “early stages” of adopting digital twin processes. “We have successfully used Witness to analyse and understand discreet processes to help us to understand buffering, flow and capacity issues,” he states. “This has helped us to make significant process investment decisions and the software clearly has potential for more widespread use.” What exactly this will lead to is yet to be determined. “The ongoing drive for digitisation is opening new possibilities,” he says. “Maybe in the future we would be able to build more widespread process models. These would help us to better analyse and optimise our manufacturing system, leading us to further improve our productivity.”
One thing Perkins is sure of, though, is that the applicability of the digital twinning approach will not be limited by process complexity; it will actually lend itself to providing solutions to particularly challenging tasks. As he concludes: “Any process that involves a degree of variability, complexity or features feedback will benefit as they are not easily understood and problem solving requires far more than a spreadsheet calculation.”