With Bosch’s manufacturing operation at Wuxi identified as a “Lighthouse” of industry 4.0, Mike Farish takes a closer look the site’s data-driven developments and explores a similar beacon of progress based in the UK
The term ‘world leader’ is easy-to-use and needs more than self-estimation to justify. But in September of last year and January of this the World Economic Forum (WEF) identified 16 manufacturing operations worldwide that it considers exemplars – ‘Lighthouses’ is its own term – of best practice in data-driven ‘Fourth Industrial Revolution’ (i4.0) procedures.
Those operations are distributed globally with the US, Europe, Middle East and Asia all represented. One of those in the latter region is the Bosch Automotive Diesel Systems (RBCD) plant in Wuxi in southern Jiangsu province, China, only a short distance inland from the coast of the East China Sea. As Christophe Chapdelaine, senior vice-president manufacturing and quality management at the plant, confirms the operation is a key tier one supplier to the growing Chinese automotive manufacturing industry. “Our main products are diesel common rail systems, exhaust gas treatment components and gasoline fuel pumps, which support Chinese OEMs to fulfil emission legislation and fuel consumption limits,” he states. “We deploy a local for local strategy and serve most of the Chinese on-highway and off-road OEMs.”
Journey time
The Wuxi plant’s operations were outlined in an extensive report into i4.0 manufacturing authored on behalf the WEF by consultants McKinsey and published when the second batch of Lighthouse sites were identified this year. It states that the plant began its journey towards big data analysis in 2015, at which time operational data from the shop floor, such as machine cycle times or part failure modes, was not readily available. Instead, such data typically required a significant amount of manual collection and preprocessing. This meant, the report continued, that continuous shopfloor improvement activities were impacted by delayed data availability, sub-optimal data quality and soon-outdated static results covering only a limited period of time.
It was therefore determined by personnel at the plant that real-time data and the insights from their analysis would provide a path to better and faster decision-making and that in turn this would improve business agility, an attribute considered vital to competitiveness in the Chinese market. In order to pilot this approach the company decided to apply it first to machining, a key manufacturing procedure at the Wuxi site. Chapdelaine again confirms the details. “We use over a hundred 5-axis machining centres which are equipped with Bosch Rexroth MTX CNC systems,” he states. “We deal with special steel as the raw material for our products and the main processes are high speed milling and drilling to high precision.”
Therefore using standard tools and guided by best practice already established at other Bosch plants, the Wuxi site set out on a six-month project to set up an industrial i4.0 framework integrating newly installed machine-condition sensors with individual cutting tool information.
“Our plant stands out as we go beyond mere pilot application, but also get significant benefits from rolling out solutions to all our value streams,” says Chapdelaine of this underpinning aspect of the overall initiative. “Bosch Nexeed PPM (Production Performance Manager) can be rolled out plant wide as a standard industrial i4.0 platform for predictive maintenance or real-time process and machine condition monitoring. It is compatible with standard industrial communication protocols like OPC-DA, which enable communication between machines and IT systems.”
Three-step process
As Chapdelaine confirms the actual data gathering at machine level is comprehensive and detailed. “We installed Bosch Rexroth control to acquire data from motors and encoders with the parameters including torque, position and angle,” he states. “We also use MEMS (micro electro-mechanical system) sensors to gather vibration data.”
This set-up is aimed at gathering three distinct types of cutting tool information, which Chapdelaine enumerates: “The first is the tool pre-setting data, like tool length, radius and run-out. The second is tool life cycle, including tool grinding/re-sharpening batch, tool reached lifetime and tool change reasons. The third comprises data on tool inventory and location.”
Chapdelaine explains that appropriate analysis tools then turn this raw data into useful information to enhance manufacturing efficiencies. “We maximise tool life and minimise the tool quantity in circulation by using advanced data analytics,” he says. “All these help us to lower the tool inventory and cost and also provide relevant experts with powerful insights.” He adds that these data gathering techniques have also been supplemented by the use RFID tags.
But data gathering technology and processing power were not by themselves sufficient to achieve the required objectives. It was also necessary to bring together data analysts with machining experts. Together these different sets of expertise enabled the visualisation of the data and the development of customisable reports with increasingly powerful analyses, including diagnostic, predictive and prescriptive functions. In consequence plant personnel now have a deep understanding of cutting tool cost drivers, can identify tool types likely to sustain extended life quantities and are able to adjust inventory to future demand.
“We have domain experts and data analysts working together on the real time data,” Chapdelaine confirms. “It is an innovation for us to apply both areas of expertise to identify new areas for improvement to support our business cases and also to help provide the agility and flexibility for us to serve the highly dynamic Chinese automotive market.” The reports, as he further explains, zero in on the causes of unnecessary expense. “We generate reports like Analyse and Diagnose Tool Premature Change Root Cause, Predict Tool Consumption and Prescription: Business Case Oriented Self-adjusting Tool Change Rule (cost vs. output…),” he says. “These customised reports combine the needs of domain experts and the recommendations of data experts and are created using a visual analytics platform, which is flexible, interactive and easily accessible by each user.”
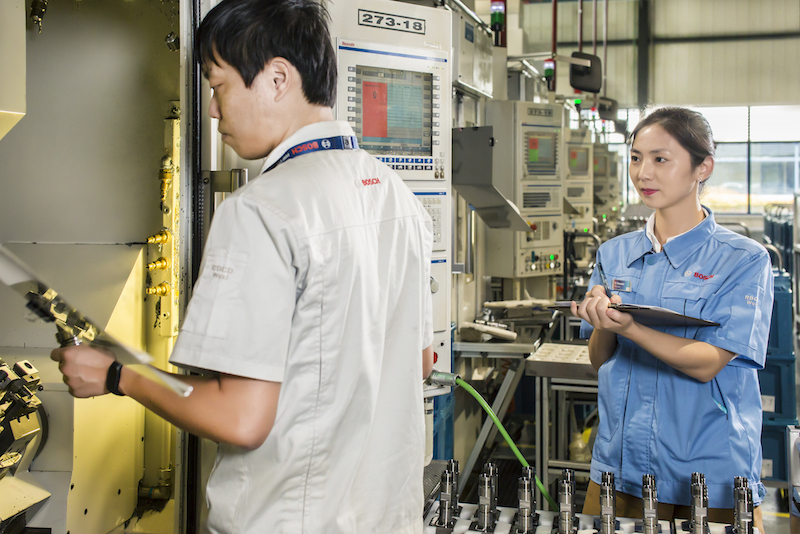
Decision-making support
At the most basic level this ‘consumption-based lean approach’ means that cutting tool inventory can be flexibly adjusted according to future demand. More fundamentally by enabling engineers to master basic data analytics in their respective domains big data analyses are supporting decision-making throughout the entire organisation as well as in manufacturing, logistics, quality management and controlling.
But it was also recognised that the identities of the individuals able to access these reports would be important and right from the start the Wuxi plant looked to introduce a holistic way of thinking that went beyond previously established divisions. The reports were therefore made available to associates and managers from all stakeholder departments in order to collect and integrate their user experience.
By mid-2017 this approach had not only led to double-digit tool cost improvements but had also inspired the organisation to come forward with further big data applications including predictive maintenance and bottleneck analysis. Over the past two years during a critical high-demand situation these activities have further contributed to an increase in output of more than ten per cent in selected areas.
Chapdelaine is in no doubt has to effectiveness of all these activities in enhancing the plant’s efficiency in wider business as well as according to internal benchmarks. ““Data analytics provides us with new methods and insights to improve quality, productivity and delivery,” he states. “All these application projects have supported the Wuxi plant in maintaining production flexibility to ensure delivery and customer satisfaction while meeting a critical high-demand situation in the China heavy-duty commercial vehicle market. At the same time they have helped reduce both the tool cost and tool inventory by two digits.”
As such Chapdelaine reiterates the need for a broad-based implementation strategy across the whole organisation. “We are applying these methods in both the technical and commercial areas,” he confirms. “This demands the development of new competences to maximise benefits and both the organisation and leadership need to evolve in order to support this transformation. This is an exciting development that both helps the speedy introduction of innovative solutions across our business to give us an edge in delivering products and services and enhances our reputation as an attractive place to work.”
Moreover further initiatives are already envisaged. “Future projects will aim at the development, integration and application of artificial intelligence, which will help bring transparency into a complex system,” Chapdelaine reports. “They will also support faster and better decision-making, innovation generation and through their requirement for new skills and technologies help attract talents in both domain and IT technologies.”

Renewing ties
A research project in the north of England featuring a company involved in the manufacture of automotive components and a local university has focussed in on the retrofitting of i4.0 technologies and data processing to improve the efficiency and effectiveness of older machine tools. The research organisation is the Advanced Manufacturing Research Centre (AMRC), part of the University of Sheffield, and the company Tinsley Bridge, a medium-sized engineering outfit employing just over 200 people based in the same city.
Russell Crow, director at Tinsley Bridge, says the company was spun out of British Steel back in the 1980s and since then has operated as a versatile manufacturing operation in different markets but with an emphasis on the supply of parts for the heavy vehicles sector. As such it acts as a tier one supplier to several of the major OEMs involved. “We supply anti-roll bars, torsion springs and stabiliser bars predominantly for the heavy good vehicles market and for some specialist military vehicles as well,” he confirms. Customers include Volvo, Iveco, Renault and Alexander Dennis with the majority of the company’s automotive output exported either to mainland Europe or the Americas. “From a single hub in Sheffield we are distributing around the world,” he states.
Last year, the company decided to investigate whether it could make more effective use of some of its existing manufacturing equipment. It chose as the test item what Crow says was “a CNC lathe about ten years old producing high-volume components.” Specifically, he continues, the company was “looking for a way to minimise the requirements on the operator in terms of quality control checks.” In effect it wanted to find a more proactive quality control technique that would be automated and predictive rather than operator-based and retrospective - “predict and prevent rather than find and fix,” as Crow puts it. The underlying business objective, he explains, was to see if it might be possible to retrofit relevant capabilities “instead of having to invest in a new machine that would have technologies like on-line probing built-in.”
In technical terms, Crow says that entailed finding a means of ensuring “non-intrusive data capture on that machine that would enable us to understand how it was operating, what the throughput was and what the quality of the output was like without burdening the operator with an inordinate amount of form-filling”. In turn that meant ensuring that the methodology employed did not in any way affect the control or operation of the machine and also minimised downtime which ruled out wiring any form of sensor technology into its controller.
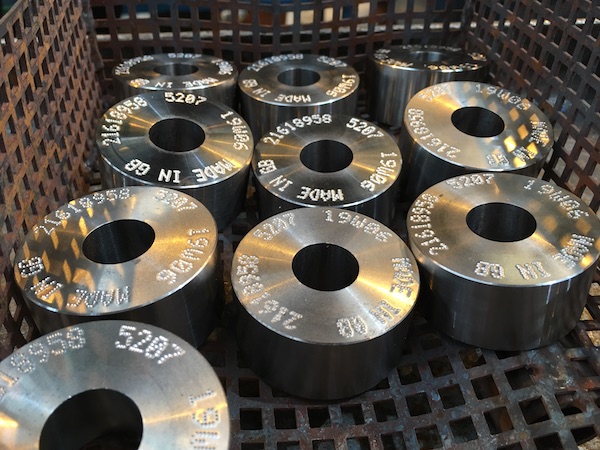
Cloud control
Crow says that the automotive part the machine was and still continues to be making is a metal boss used as a mounting point for a stabiliser bar. “It is effectively a metal cylinder that contains a conical taper for connection to a droplink so the fit and function make it a safety-critical part,” he states. As such continuous operator supervision is an absolute requirement of the manufacturing process, which is carried out on a just-in-time basis.
A simple means of monitoring the machine’s operations was soon found – fixing basic ‘current clamps’ to the power cable to the machine’s cutting head which recorded the varying power consumption during machining operations. The question then was how could this basic data be processed in a way that might generate useful information.
This was where the AMRC became closely involved. It used its specialist expertise to upload the data to the Cloud and then fed it into an artificial intelligence (AI) computer program running at its campus site base. “They had developed an algorithm that could discern between different types of part on the machine and pick up on variations in signature for parts of the same type,” confirms Crow. “We will develop this into a real time monitor of the process variables that engages the company’s zero defect quality philosophy.”
Crow is confident that the project has demonstrated the feasibility of coupling i4.0 techniques of continuous sensing, internet-based communications and AI software programming with shopfloor data capture to support machining operations using relatively unsophisticated equipment. Moreover the data capture elements were also straightforward and inexpensive – the data was uploaded from the machine using the well-known MQTT protocol while the AI software ran on a PC hardware platform. As such he feels that the project has laid the foundations for further exploration of how this combination of technologies can be utilised in a more proactive manner to support real manufacturing operations: “We can now get shopfloor data capture that lets us know how that asset is performing. The next step is to explore how to use that data as a planning tool.”
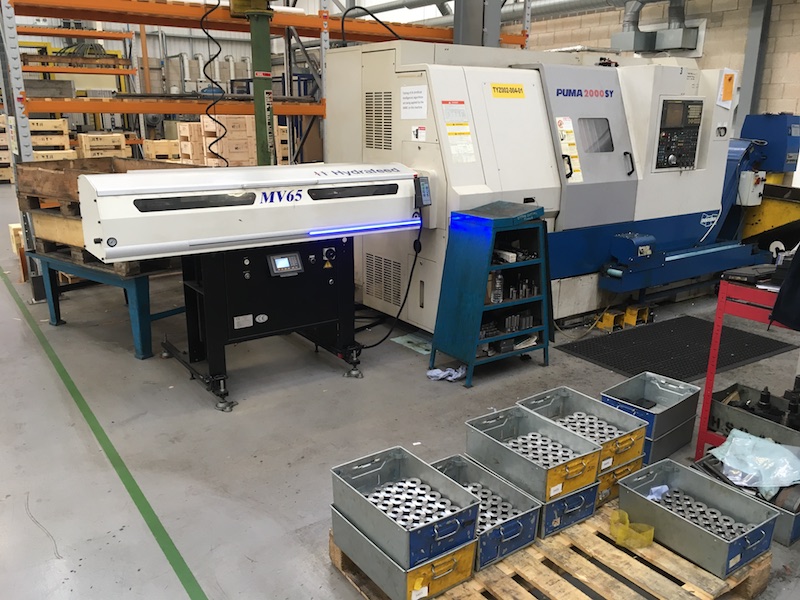